Have you encountered the following problems when using AI?
- Limited context windowLLM cannot understand and analyze large amounts of information.
- Knowledge is scattered and difficult to integrateThe knowledge information within the organization is often scattered in various systems or documents, and it is difficult to coordinate and manage.
- LLM lacks specific domain knowledgeLLM does not have the knowledge reserve of a specific team, organization, enterprise or industry, and cannot respond.
Rich sources and forms of knowledge
Strong knowledge integration ability
It provides a variety of ways to add knowledge, supports uploading of various document formats (doc/pdf/md/txt/csv/xls/...), and also supports crawling website content as knowledge, gathering scattered knowledge data together.
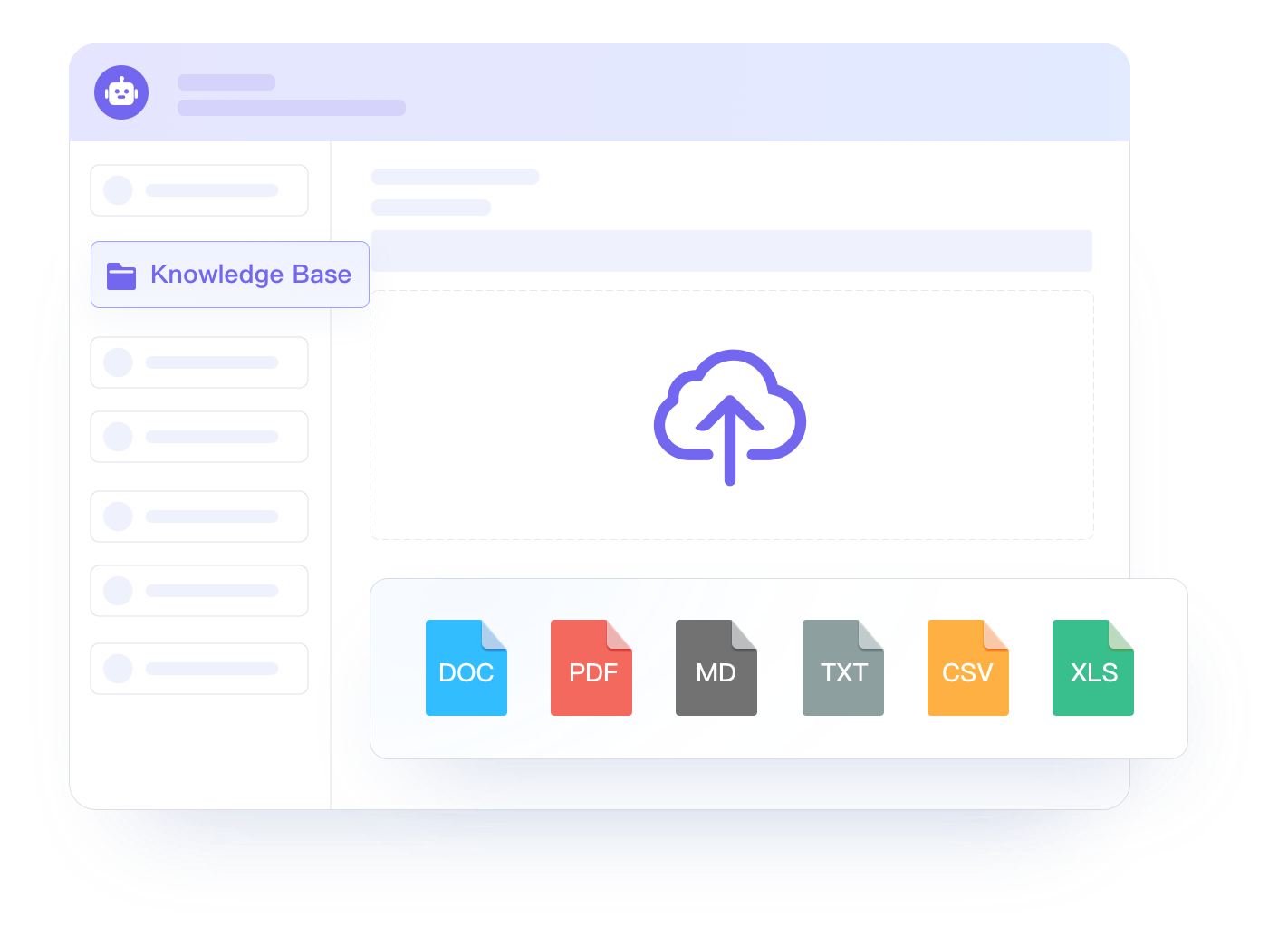
Structured data and unstructured data
In addition to unstructured data (such as: traditional documents), it also supports structured data (tables, Q&A) as knowledge data, allowing all types of data to be efficiently retrieved.
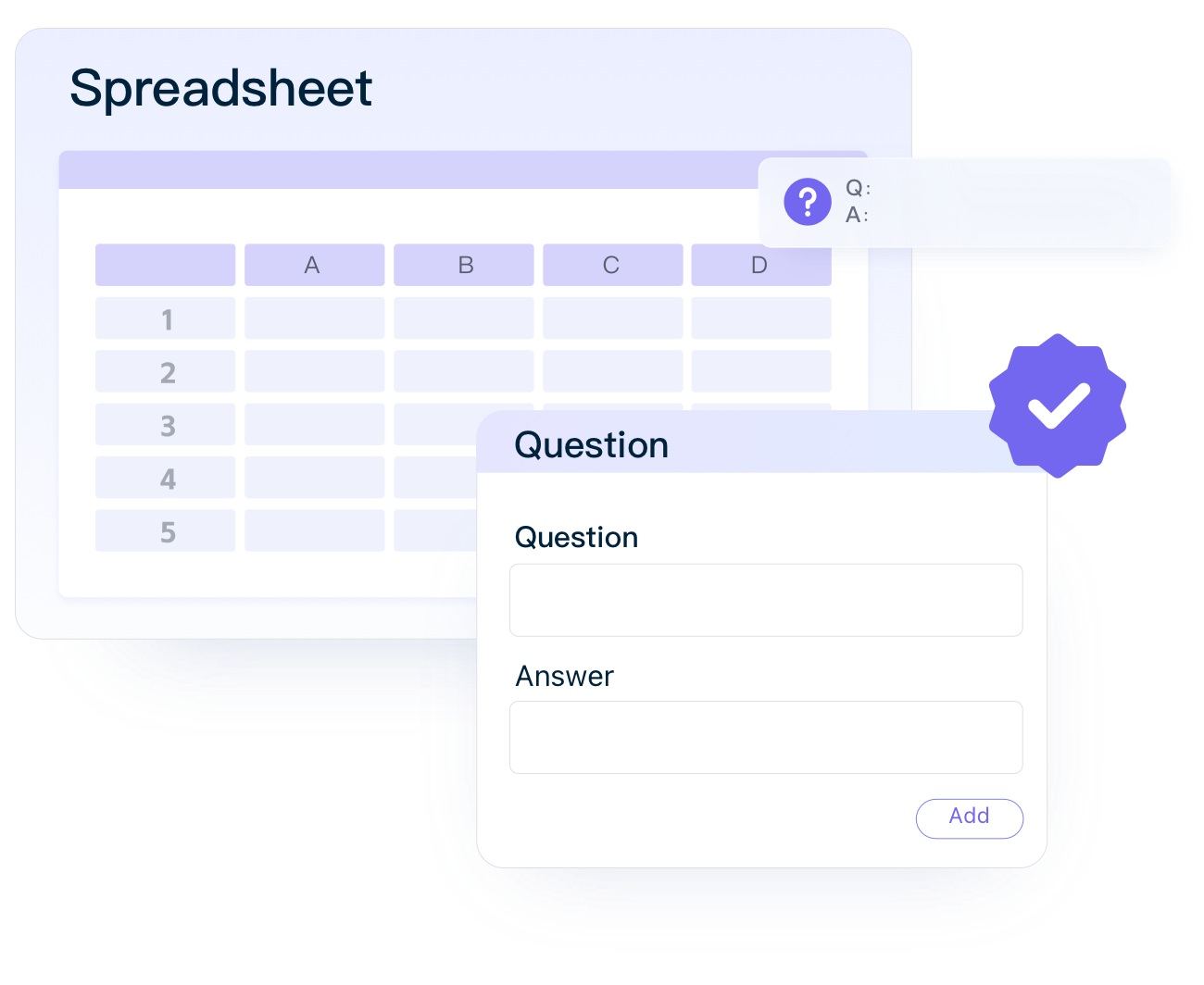
Third-party applications and API
Supports adding knowledge through third-party applications (Notion, Dropbox, Google Drive, ……), and also supports adding knowledge through API, so that private knowledge stored in third-party applications can be easily imported.
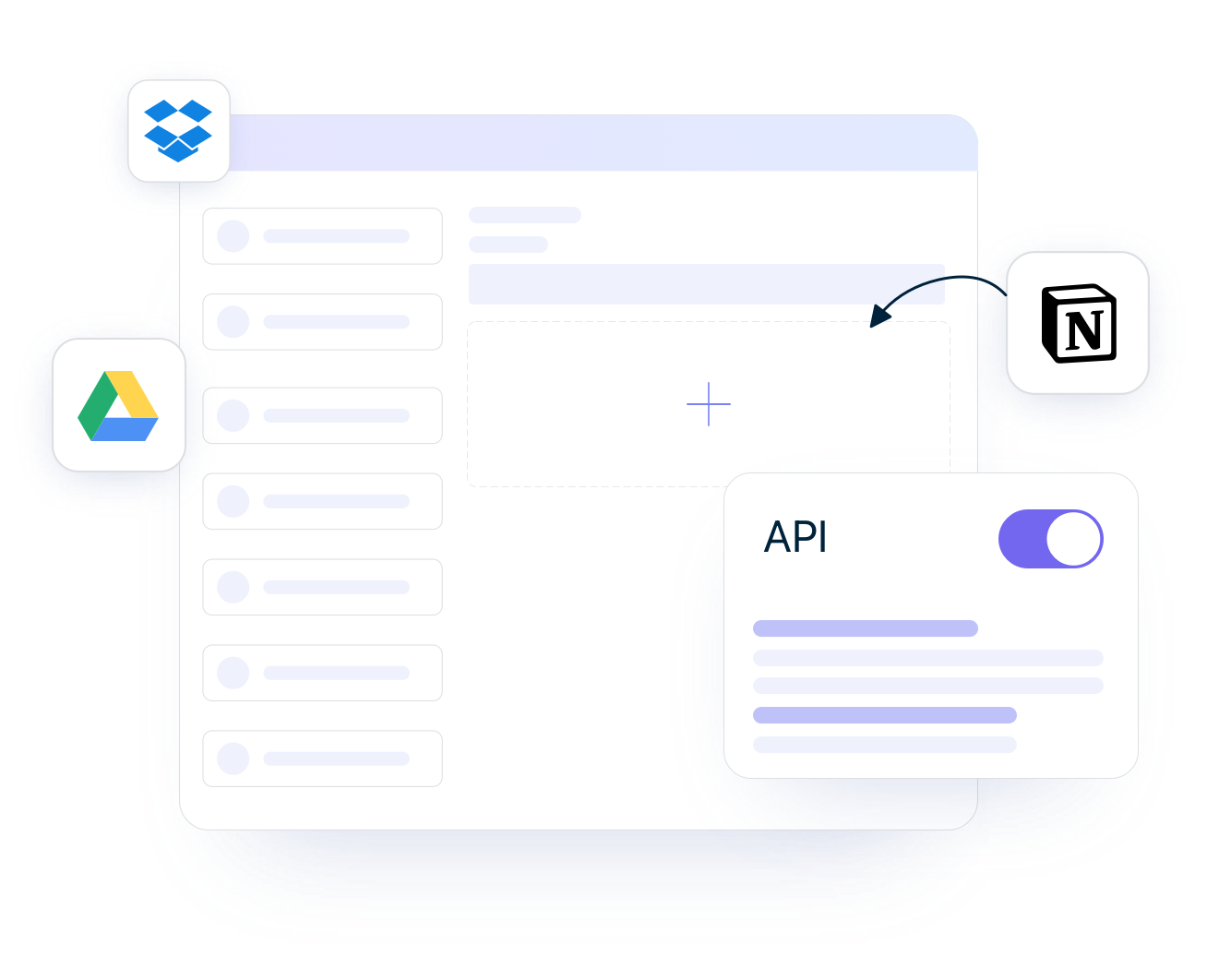
Easy-to-operate knowledge management module
Knowledge online editing
Need to modify the uploaded knowledge? Just save it directly after online editing, no need to modify it offline and resubmit it.Web page knowledge update
Web page information often changes? One click can re-crawl the page information and update it to the knowledge base, no need to re-add.Knowledge slice management
It provides a flexible slicing method, which allows knowledge fragments to be managed more reasonably. Support adding, modifying and deleting knowledge slices, making knowledge management more detailed and flexible.
RAG: Strong knowledge embedding, retrieval and recall capabilities
High-quality knowledge embedding model
Access to the world's top embedding model, making semantic matching of knowledge more accurate and reasonable.Mixed retrieval mode
Exclusive development of "dense vector + sparse vector" mixed retrieval mode, taking into account "vector retrieval" and traditional "keyword retrieval", allowing retrieval to get more accurate recall results in various scenarios.Vector retrieval test
Not sure how the knowledge retrieval effect is? Direct retrieval test, observe the recalled knowledge slices and relevance, intuitively understand the retrieval results, and optimize the Bot configuration and knowledge data reasonably based on this.
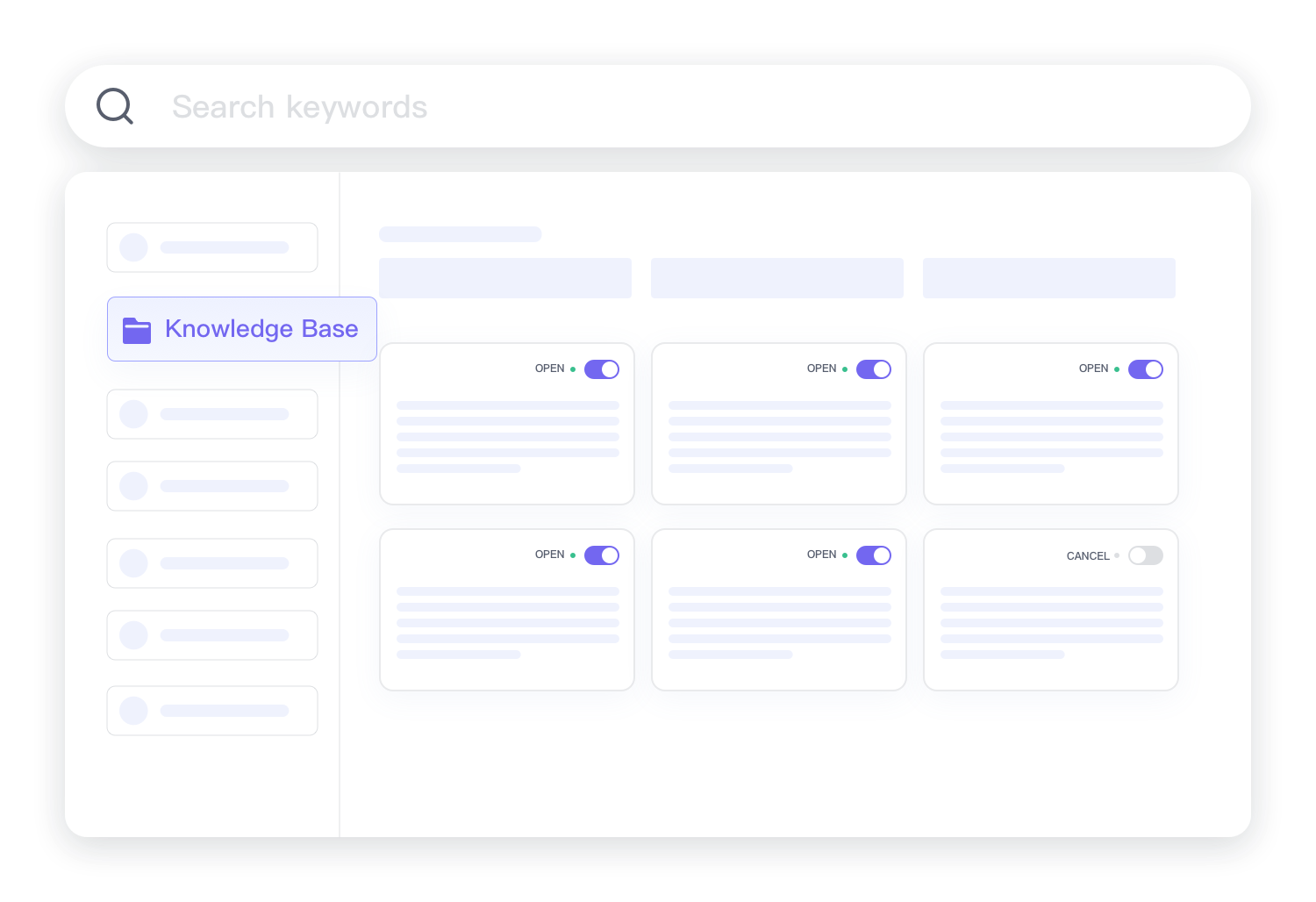
Make knowledge more valuable
The best knowledge structure
The knowledge form that best conforms to the RAG architecture knowledge recall and LLM fine-tuning, supports online adding and editing, and also supports intelligently converting articles into multiple "Q&A".
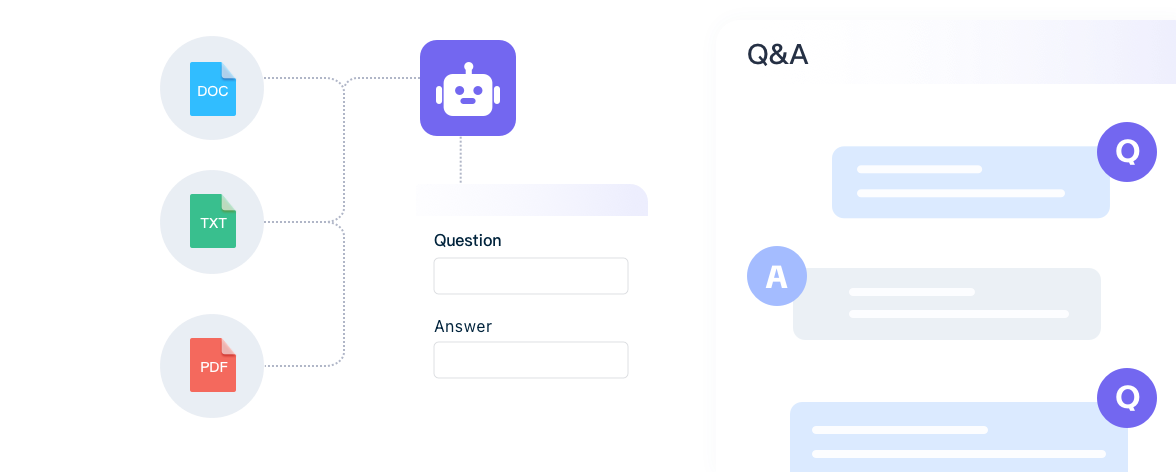
More accurate knowledge recall
The structured "Q&A" storage method is more conducive to accurate recall of knowledge.
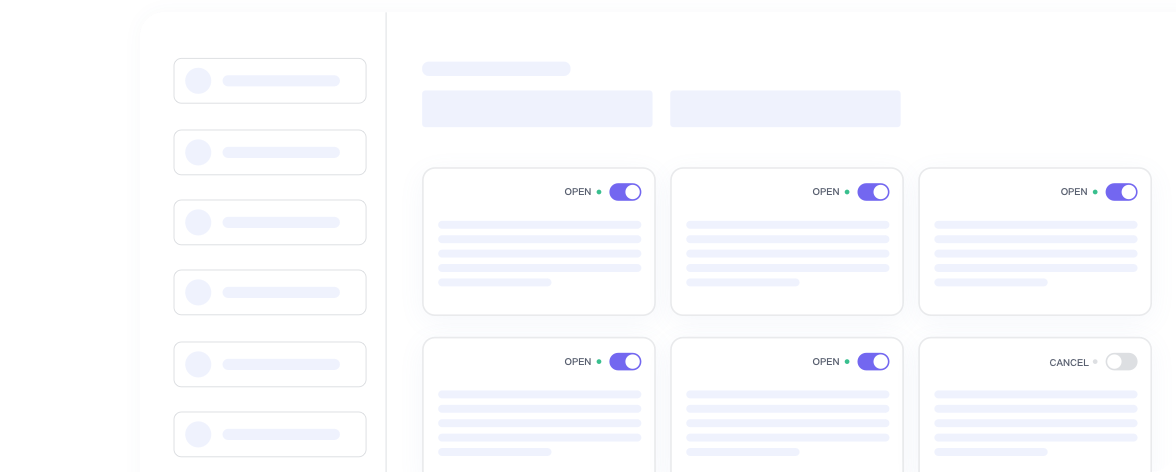
Efficient training for Bot
Use real chat history records, provide feedback, and precipitate the knowledge in the chat record into "Q&A" to improve Bot, so that Bot can improve in each interaction.
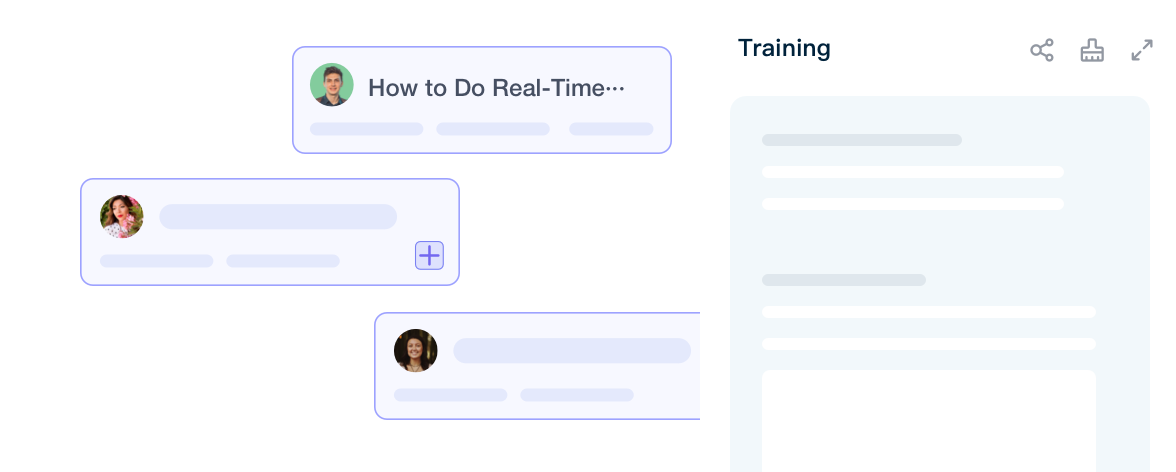
Make LLM fine-tuning easier
The "Q&A" structured knowledge can be directly used in LLM fine-tuning, which makes the fine-tuning work easier.
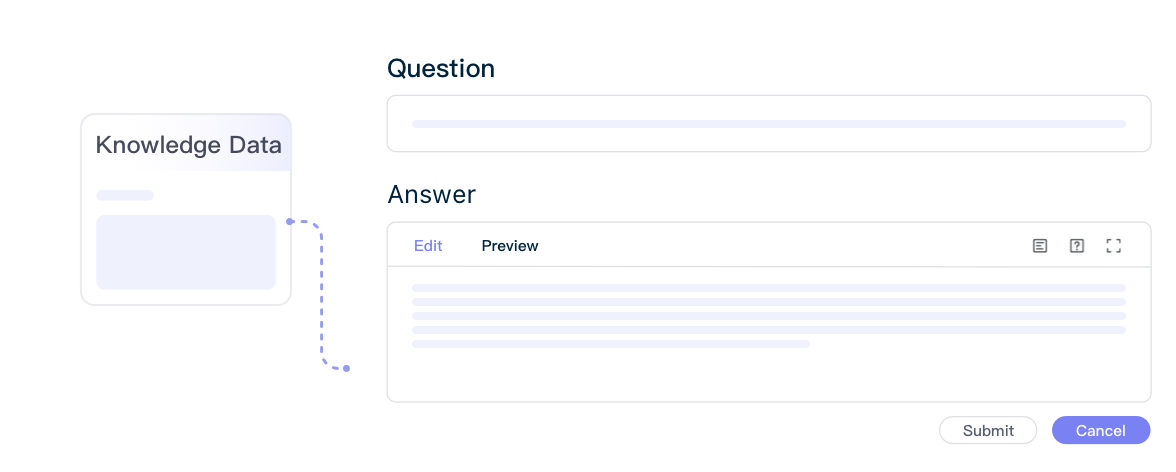
Knowledge data storage, safe and compliant
Protecting your data is our top priority!
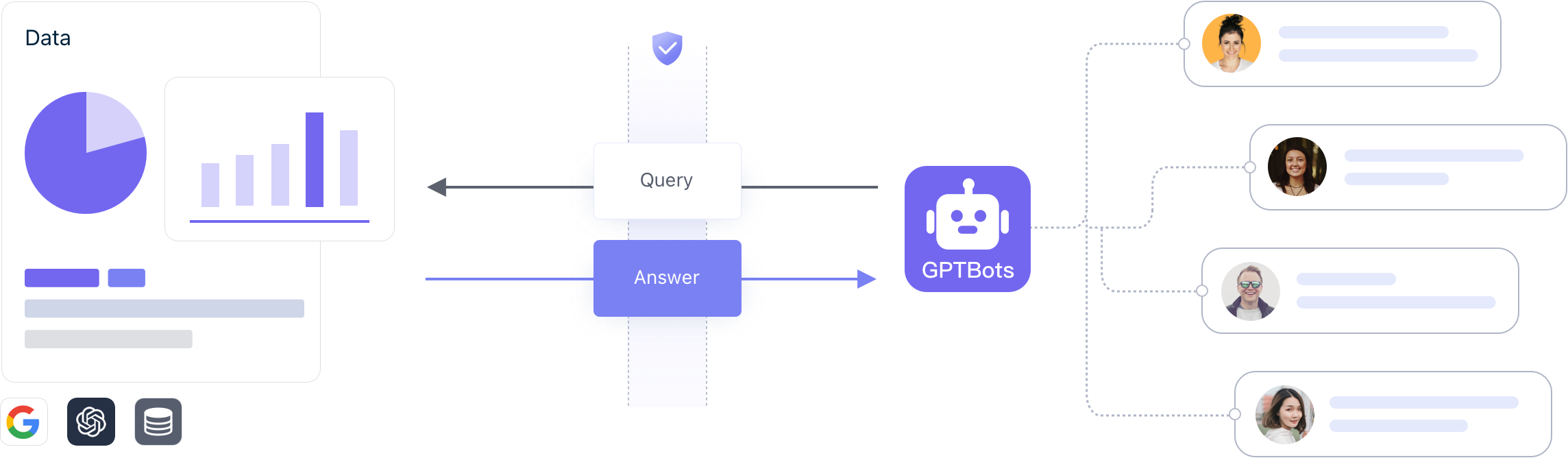
GPTBots uses advanced technologies such as transmission encryption, security encryption and account data isolation to ensure that your data is always in a highly secure state. Through multiple backups and reliable cloud platforms, we provide you with rock-solid data security. Choose us, trust and peace of mind are with you.
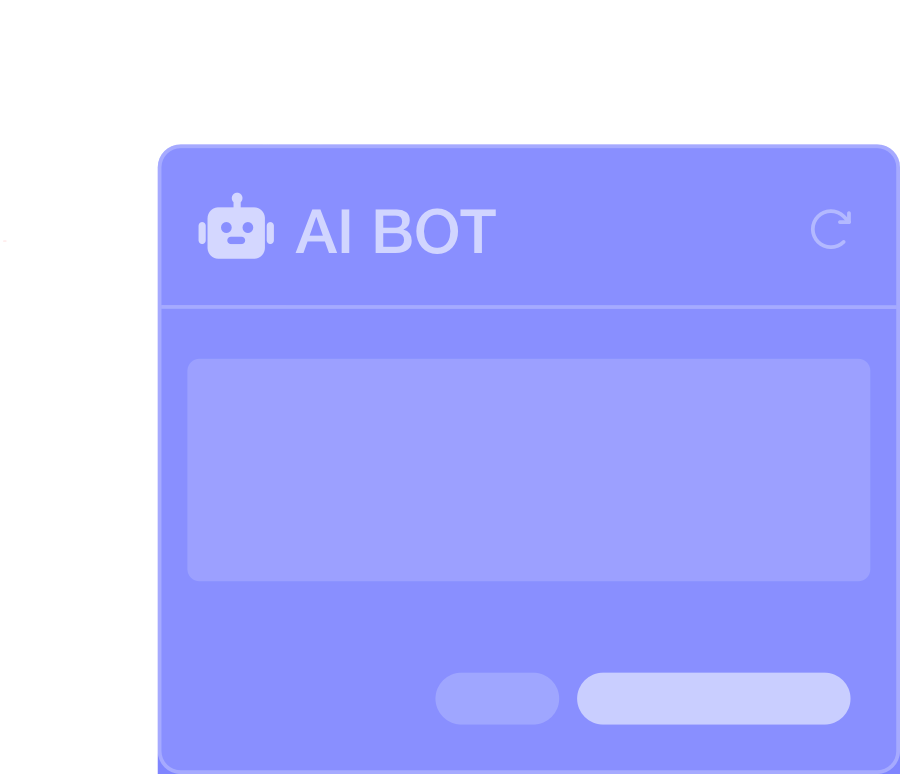